Sorting, heads and tails#
# Load the Pandas data science library, call it 'pd'
import pandas as pd
# Turn on a setting to use Pandas more safely.
# We will discuss this setting later.
pd.set_option('mode.copy_on_write', True)
# Load the library for plotting, name it 'plt'
import matplotlib.pyplot as plt
# Make plots look a little more fancy
plt.style.use('fivethirtyeight')
In basic column indexing we found the slightly odd result that, for rich countries, there is little relationship between GDP and maternal mortality.
Here we investigate further, by looking at rich and poor countries separately.
In order to do that, we are going to sort our DataFrame using the
.sort_values
method, and the select the first and last group of rows, using
the .head
and .tail
methods.
First let us return to the slightly processed DataFrame we were working on before:
# Original data frame before dropping missing values.
gender_data = pd.read_csv('gender_stats_min.csv')
gender_data_no_na = gender_data.dropna()
labeled_gdata = gender_data_no_na.set_index('country_code')
labeled_gdata
country_name | gdp_us_billion | mat_mort_ratio | population | |
---|---|---|---|---|
country_code | ||||
AFG | Afghanistan | 19.961015 | 444.00 | 32.715838 |
AGO | Angola | 111.936542 | 501.25 | 26.937545 |
ALB | Albania | 12.327586 | 29.25 | 2.888280 |
ARE | United Arab Emirates | 375.027082 | 6.00 | 9.080299 |
ARG | Argentina | 550.980968 | 53.75 | 42.976675 |
... | ... | ... | ... | ... |
WSM | Samoa | 0.799887 | 54.75 | 0.192225 |
YEM | Yemen, Rep. | 36.819337 | 399.75 | 26.246608 |
ZAF | South Africa | 345.209888 | 143.75 | 54.177209 |
ZMB | Zambia | 24.280990 | 233.75 | 15.633220 |
ZWE | Zimbabwe | 15.495514 | 398.00 | 15.420964 |
179 rows × 4 columns
Here is the plot we saw before, with the unconvincing relationship of GDP to Maternal Mortality Rate (MMR).
plt.scatter(labeled_gdata['gdp_us_billion'],
labeled_gdata['mat_mort_ratio'])
plt.title('MMR as a function of GDP')
Text(0.5, 1.0, 'MMR as a function of GDP')
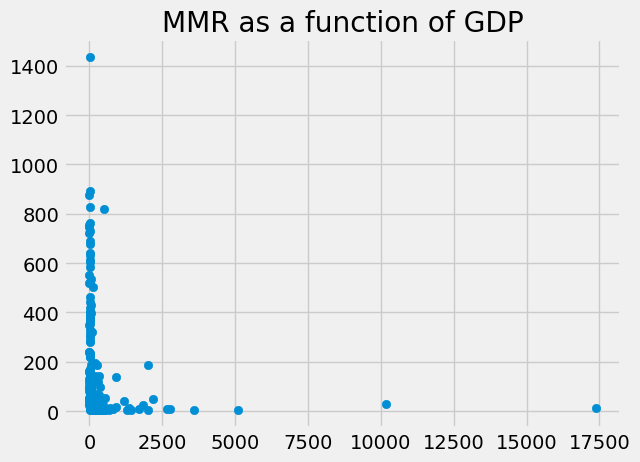
We wondered whether the relationship of GDP and MMR might be different for rich and poor countries.
To look at that, we can sort the DataFrame by the GDP values.
In order to do that, we use the .sort_values
method, passing the column name containing the values we want to sort by:
gdata_by_gdp = labeled_gdata.sort_values('gdp_us_billion')
gdata_by_gdp
country_name | gdp_us_billion | mat_mort_ratio | population | |
---|---|---|---|---|
country_code | ||||
KIR | Kiribati | 0.177431 | 95.00 | 0.110482 |
STP | Sao Tome and Principe | 0.314540 | 159.50 | 0.191333 |
FSM | Micronesia, Fed. Sts. | 0.319321 | 103.25 | 0.104118 |
TON | Tonga | 0.439179 | 129.25 | 0.105909 |
COM | Comoros | 0.603919 | 349.50 | 0.759556 |
... | ... | ... | ... | ... |
GBR | United Kingdom | 2768.864417 | 9.25 | 64.641557 |
DEU | Germany | 3601.226158 | 6.25 | 81.281645 |
JPN | Japan | 5106.024760 | 5.75 | 127.297102 |
CHN | China | 10182.790479 | 28.75 | 1364.446000 |
USA | United States | 17369.124600 | 14.00 | 318.558175 |
179 rows × 4 columns
Notice that the .sort_values
method returned a new data frame with the rows
in ascending order of the values in the given column (here gdp_us_billion
).
We therefore have a DataFrame where the richest countries are first and the
poorest last.
Ascending order is the default sort order, but you can ask for descending order by giving the ascending
keyword argument a value of False
, like this:
gdata_by_desc_gdp = labeled_gdata.sort_values('gdp_us_billion',
ascending=False)
gdata_by_desc_gdp
country_name | gdp_us_billion | mat_mort_ratio | population | |
---|---|---|---|---|
country_code | ||||
USA | United States | 17369.124600 | 14.00 | 318.558175 |
CHN | China | 10182.790479 | 28.75 | 1364.446000 |
JPN | Japan | 5106.024760 | 5.75 | 127.297102 |
DEU | Germany | 3601.226158 | 6.25 | 81.281645 |
GBR | United Kingdom | 2768.864417 | 9.25 | 64.641557 |
... | ... | ... | ... | ... |
COM | Comoros | 0.603919 | 349.50 | 0.759556 |
TON | Tonga | 0.439179 | 129.25 | 0.105909 |
FSM | Micronesia, Fed. Sts. | 0.319321 | 103.25 | 0.104118 |
STP | Sao Tome and Principe | 0.314540 | 159.50 | 0.191333 |
KIR | Kiribati | 0.177431 | 95.00 | 0.110482 |
179 rows × 4 columns
Notice that now the richest countries are first and the poorest last.
Let us go back to the poorest to richest sorted DataFrame, gdata_by_gdp
. The DataFrame has a .head
method that, by default, will select the first 5 rows of the DataFrame:
gdata_by_gdp.head()
country_name | gdp_us_billion | mat_mort_ratio | population | |
---|---|---|---|---|
country_code | ||||
KIR | Kiribati | 0.177431 | 95.00 | 0.110482 |
STP | Sao Tome and Principe | 0.314540 | 159.50 | 0.191333 |
FSM | Micronesia, Fed. Sts. | 0.319321 | 103.25 | 0.104118 |
TON | Tonga | 0.439179 | 129.25 | 0.105909 |
COM | Comoros | 0.603919 | 349.50 | 0.759556 |
Notice that the result is a new DataFrame that only has 5 rows.
In fact we often use .head
to show a small sample of the DataFrame, and you will see that use throughout the rest of the course.
You can also give .head
a number of rows you want. For example to select the
125 poorest countries (in terms of GDP), you could use:
poorest_125 = gdata_by_gdp.head(125)
poorest_125
country_name | gdp_us_billion | mat_mort_ratio | population | |
---|---|---|---|---|
country_code | ||||
KIR | Kiribati | 0.177431 | 95.00 | 0.110482 |
STP | Sao Tome and Principe | 0.314540 | 159.50 | 0.191333 |
FSM | Micronesia, Fed. Sts. | 0.319321 | 103.25 | 0.104118 |
TON | Tonga | 0.439179 | 129.25 | 0.105909 |
COM | Comoros | 0.603919 | 349.50 | 0.759556 |
... | ... | ... | ... | ... |
AGO | Angola | 111.936542 | 501.25 | 26.937545 |
HUN | Hungary | 129.470864 | 16.25 | 9.868180 |
UKR | Ukraine | 135.379275 | 24.25 | 45.302704 |
KWT | Kuwait | 156.226123 | 4.00 | 3.752954 |
BGD | Bangladesh | 174.545099 | 194.75 | 159.371214 |
125 rows × 4 columns
Now we have the rows corresponding to the 125 poorest countries, we can repeat our GDP / MMR plot, restricted to those countries:
plt.scatter(poorest_125['gdp_us_billion'], poorest_125['mat_mort_ratio'])
plt.title('MMR as a function of GDP, for 125 poorest countries')
Text(0.5, 1.0, 'MMR as a function of GDP, for 125 poorest countries')
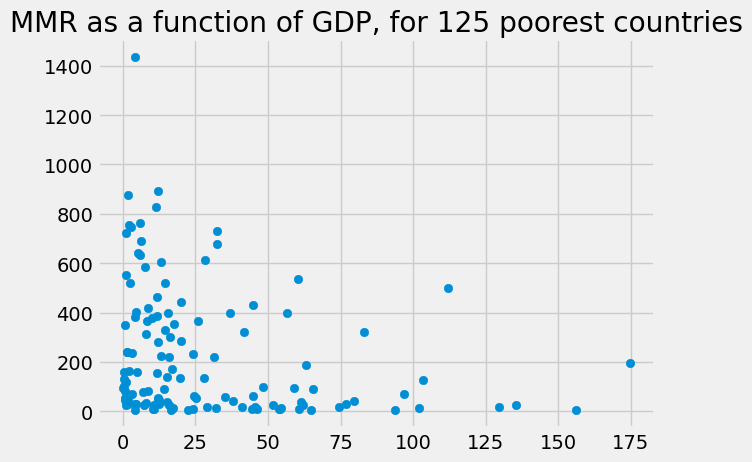
If we sort the new DataFrame by the MMR values, we can see which of these 125 poorest countries are doing particularly well or badly in terms of MMR:
poorest_125.sort_values('mat_mort_ratio')
country_name | gdp_us_billion | mat_mort_ratio | population | |
---|---|---|---|---|
country_code | ||||
ISL | Iceland | 16.741585 | 3.50 | 0.327387 |
BLR | Belarus | 64.782942 | 4.00 | 9.480348 |
KWT | Kuwait | 156.226123 | 4.00 | 3.752954 |
SVK | Slovak Republic | 93.894473 | 6.00 | 5.418425 |
CYP | Cyprus | 22.347398 | 7.00 | 1.152475 |
... | ... | ... | ... | ... |
SOM | Somalia | 5.785250 | 762.75 | 13.527075 |
SSD | South Sudan | 11.480939 | 827.50 | 11.527917 |
CAF | Central African Republic | 1.749110 | 875.75 | 4.529236 |
TCD | Chad | 11.945942 | 892.25 | 13.574024 |
SLE | Sierra Leone | 4.331604 | 1435.00 | 7.080112 |
125 rows × 4 columns
DataFrames also have .tail
method that, by default, gives the last 5 rows of the DataFrame. For example, these are the 5 richest countries:
gdata_by_gdp.tail()
country_name | gdp_us_billion | mat_mort_ratio | population | |
---|---|---|---|---|
country_code | ||||
GBR | United Kingdom | 2768.864417 | 9.25 | 64.641557 |
DEU | Germany | 3601.226158 | 6.25 | 81.281645 |
JPN | Japan | 5106.024760 | 5.75 | 127.297102 |
CHN | China | 10182.790479 | 28.75 | 1364.446000 |
USA | United States | 17369.124600 | 14.00 | 318.558175 |
Like .head
we can give .tail
a number of rows we want. Here we are looking at the last 25 rows of the sorted DataFrame, and therefore, the 25 richest countries:
richest_25 = gdata_by_gdp.tail(25)
richest_25
country_name | gdp_us_billion | mat_mort_ratio | population | |
---|---|---|---|---|
country_code | ||||
NGA | Nigeria | 486.113579 | 818.50 | 176.551695 |
BEL | Belgium | 494.221836 | 7.00 | 11.228495 |
POL | Poland | 503.311262 | 3.00 | 38.009905 |
SWE | Sweden | 540.626904 | 4.00 | 9.703634 |
ARG | Argentina | 550.980968 | 53.75 | 42.976675 |
CHE | Switzerland | 676.642359 | 5.25 | 8.185870 |
SAU | Saudi Arabia | 707.936120 | 12.25 | 30.728077 |
NLD | Netherlands | 819.285000 | 7.00 | 16.876547 |
TUR | Turkey | 895.175577 | 17.50 | 77.034345 |
IDN | Indonesia | 902.944866 | 136.75 | 255.064836 |
MEX | Mexico | 1188.802780 | 40.00 | 124.203450 |
ESP | Spain | 1299.724261 | 5.00 | 46.553128 |
KOR | Korea, Rep. | 1346.751162 | 12.00 | 50.727212 |
AUS | Australia | 1422.994116 | 6.00 | 23.444560 |
CAN | Canada | 1708.473627 | 7.25 | 35.517119 |
RUS | Russian Federation | 1822.691700 | 25.25 | 143.793504 |
ITA | Italy | 2005.983980 | 4.00 | 60.378795 |
IND | India | 2019.005411 | 185.25 | 1293.742537 |
BRA | Brazil | 2198.765606 | 49.50 | 204.159544 |
FRA | France | 2647.649725 | 8.75 | 66.302099 |
GBR | United Kingdom | 2768.864417 | 9.25 | 64.641557 |
DEU | Germany | 3601.226158 | 6.25 | 81.281645 |
JPN | Japan | 5106.024760 | 5.75 | 127.297102 |
CHN | China | 10182.790479 | 28.75 | 1364.446000 |
USA | United States | 17369.124600 | 14.00 | 318.558175 |
plt.scatter(richest_25['gdp_us_billion'], richest_25['mat_mort_ratio'])
plt.title('MMR as a function of GDP, for 25 richest countries')
Text(0.5, 1.0, 'MMR as a function of GDP, for 25 richest countries')
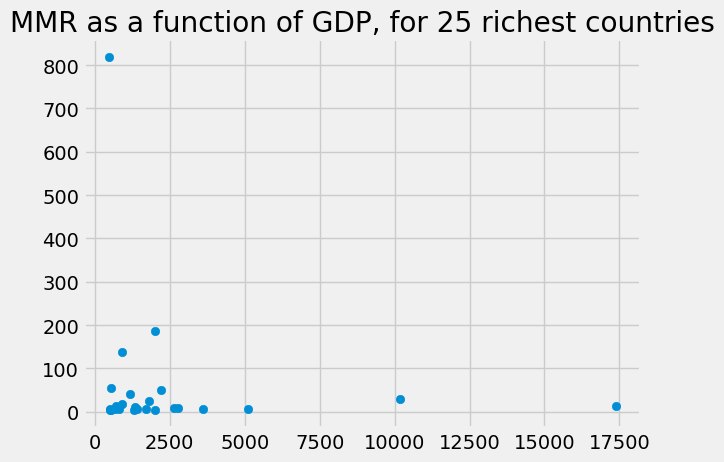
Again, we can sort by the MMR values to show the best and worst countries in terms of maternal health:
richest_25.sort_values('mat_mort_ratio')
country_name | gdp_us_billion | mat_mort_ratio | population | |
---|---|---|---|---|
country_code | ||||
POL | Poland | 503.311262 | 3.00 | 38.009905 |
SWE | Sweden | 540.626904 | 4.00 | 9.703634 |
ITA | Italy | 2005.983980 | 4.00 | 60.378795 |
ESP | Spain | 1299.724261 | 5.00 | 46.553128 |
CHE | Switzerland | 676.642359 | 5.25 | 8.185870 |
JPN | Japan | 5106.024760 | 5.75 | 127.297102 |
AUS | Australia | 1422.994116 | 6.00 | 23.444560 |
DEU | Germany | 3601.226158 | 6.25 | 81.281645 |
BEL | Belgium | 494.221836 | 7.00 | 11.228495 |
NLD | Netherlands | 819.285000 | 7.00 | 16.876547 |
CAN | Canada | 1708.473627 | 7.25 | 35.517119 |
FRA | France | 2647.649725 | 8.75 | 66.302099 |
GBR | United Kingdom | 2768.864417 | 9.25 | 64.641557 |
KOR | Korea, Rep. | 1346.751162 | 12.00 | 50.727212 |
SAU | Saudi Arabia | 707.936120 | 12.25 | 30.728077 |
USA | United States | 17369.124600 | 14.00 | 318.558175 |
TUR | Turkey | 895.175577 | 17.50 | 77.034345 |
RUS | Russian Federation | 1822.691700 | 25.25 | 143.793504 |
CHN | China | 10182.790479 | 28.75 | 1364.446000 |
MEX | Mexico | 1188.802780 | 40.00 | 124.203450 |
BRA | Brazil | 2198.765606 | 49.50 | 204.159544 |
ARG | Argentina | 550.980968 | 53.75 | 42.976675 |
IDN | Indonesia | 902.944866 | 136.75 | 255.064836 |
IND | India | 2019.005411 | 185.25 | 1293.742537 |
NGA | Nigeria | 486.113579 | 818.50 | 176.551695 |
To investigate further, we need to do some calculations to adjust for the population.