Selecting rows from DataFrames#
# Load the Pandas data science library, call it 'pd'
import pandas as pd
# Turn on a setting to use Pandas more safely.
# We will discuss this setting later.
pd.set_option('mode.copy_on_write', True)
# Load the library for plotting, name it 'plt'
import matplotlib.pyplot as plt
# Make plots look a little more fancy
plt.style.use('fivethirtyeight')
We return again to the DataFrame we were working on in basic column indexing.
# Original data frame before dropping missing values.
gender_data = pd.read_csv('gender_stats_min.csv')
labeled_gdata = gender_data.dropna().set_index('country_code')
# Show the result
labeled_gdata
country_name | gdp_us_billion | mat_mort_ratio | population | |
---|---|---|---|---|
country_code | ||||
AFG | Afghanistan | 19.961015 | 444.00 | 32.715838 |
AGO | Angola | 111.936542 | 501.25 | 26.937545 |
ALB | Albania | 12.327586 | 29.25 | 2.888280 |
ARE | United Arab Emirates | 375.027082 | 6.00 | 9.080299 |
ARG | Argentina | 550.980968 | 53.75 | 42.976675 |
... | ... | ... | ... | ... |
WSM | Samoa | 0.799887 | 54.75 | 0.192225 |
YEM | Yemen, Rep. | 36.819337 | 399.75 | 26.246608 |
ZAF | South Africa | 345.209888 | 143.75 | 54.177209 |
ZMB | Zambia | 24.280990 | 233.75 | 15.633220 |
ZWE | Zimbabwe | 15.495514 | 398.00 | 15.420964 |
179 rows × 4 columns
Thus far we have used DataFrame indexing to select columns from DataFrames.
Remember, indexing is where we use square brackets following a value in order to select data from inside that value.
We also often want to select rows from DataFrames. You have already seen
the .head
and .tail
methods, for selecting rows.
This page covers a more general, and more useful way of selecting rows, using direct indexing with a Boolean Series (DIBS).
Direct indexing with a Boolean Series#
We often want to select rows from the data frame that match some criterion.
For example, let us return to the problem of comparing richer and poorer
countries. So far we have sorted the DataFrame with .sort_values
and then
selected the first \(n\) or last \(n\) values, using .head
and .tail
. But what
if we wanted to select the rows corresponding the countries with a GDP above
some threshold.
Here’s are the GDP values as a Series. We are using direct indexing with column labels (DICL) on the DataFrame, to get the column as a Series:
# Direct indexing with column labels (DICL).
gdp = labeled_gdata['gdp_us_billion']
gdp
country_code
AFG 19.961015
AGO 111.936542
ALB 12.327586
ARE 375.027082
ARG 550.980968
...
WSM 0.799887
YEM 36.819337
ZAF 345.209888
ZMB 24.280990
ZWE 15.495514
Name: gdp_us_billion, Length: 179, dtype: float64
Here’s the histogram of GDP again:
plt.hist(gdp)
(array([169., 6., 2., 0., 0., 1., 0., 0., 0., 1.]),
array([1.77430636e-01, 1.73707215e+03, 3.47396686e+03, 5.21086158e+03,
6.94775630e+03, 8.68465102e+03, 1.04215457e+04, 1.21584404e+04,
1.38953352e+04, 1.56322299e+04, 1.73691246e+04]),
<BarContainer object of 10 artists>)
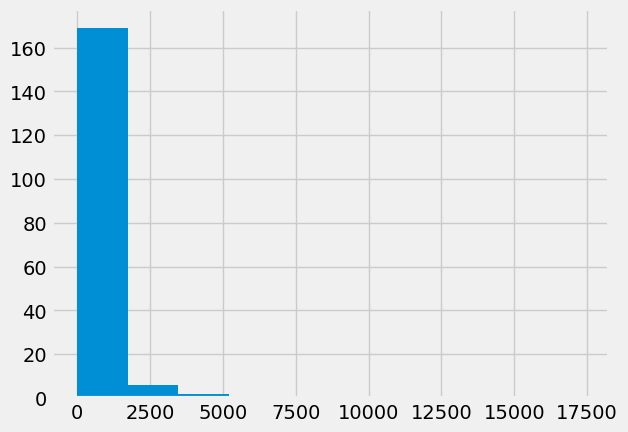
Looking at the histogram, we could try 1000 (billion US dollars) as a threshold to identify high GDP countries.
As you have found in the Series are like arrays page,
Series are like arrays in many ways. As you remember, if you do a comparison
on an array, you get a Boolean array, where each value in the Boolean array is
the True
or False
answer to the comparison question for the corresponding
element in the original array. If you do a comparison on a Series, you get a
Boolean Series, where each value in the Series is the True
or False
answer
to the comparison question for the corresponding element in the original
Series.
For example, here we do a comparison on the gdp
series, and we get a Boolean Series.
gdp_gt_1000 = gdp > 1000
gdp_gt_1000
country_code
AFG False
AGO False
ALB False
ARE False
ARG False
...
WSM False
YEM False
ZAF False
ZMB False
ZWE False
Name: gdp_us_billion, Length: 179, dtype: bool
Notice that the Boolean Series has the same row labels (index) as the original
Series (here, gdp
).
The Boolean Series is — a Series:
type(gdp_gt_1000)
pandas.core.series.Series
We can use this Boolean series to select rows from the DataFrame, by Boolean indexing — direct indexing with Boolean Series (DIBS).
When we index using the Boolean Series inside the square brackets, it works like as it does when we index an array with a Boolean array:
rich_gender_data = labeled_gdata[gdp_gt_1000]
rich_gender_data
country_name | gdp_us_billion | mat_mort_ratio | population | |
---|---|---|---|---|
country_code | ||||
AUS | Australia | 1422.994116 | 6.00 | 23.444560 |
BRA | Brazil | 2198.765606 | 49.50 | 204.159544 |
CAN | Canada | 1708.473627 | 7.25 | 35.517119 |
CHN | China | 10182.790479 | 28.75 | 1364.446000 |
DEU | Germany | 3601.226158 | 6.25 | 81.281645 |
ESP | Spain | 1299.724261 | 5.00 | 46.553128 |
FRA | France | 2647.649725 | 8.75 | 66.302099 |
GBR | United Kingdom | 2768.864417 | 9.25 | 64.641557 |
IND | India | 2019.005411 | 185.25 | 1293.742537 |
ITA | Italy | 2005.983980 | 4.00 | 60.378795 |
JPN | Japan | 5106.024760 | 5.75 | 127.297102 |
KOR | Korea, Rep. | 1346.751162 | 12.00 | 50.727212 |
MEX | Mexico | 1188.802780 | 40.00 | 124.203450 |
RUS | Russian Federation | 1822.691700 | 25.25 | 143.793504 |
USA | United States | 17369.124600 | 14.00 | 318.558175 |
type(rich_gender_data)
pandas.core.frame.DataFrame
rich_gender_data
is a new data frame, that is a subset of the original
labeled_gdata
frame. It contains only the rows where the GDP value is
greater than 1000
billion dollars. Check the display of rich_gender_data
above to confirm that the values in the gdp_us_billion
column are all greater
than 1000
.
We can do a scatter plot of GDP values against maternal mortality rate, and we find again that, for rich countries, there is little relationship between GDP and maternal mortality.
plt.scatter(rich_gender_data['gdp_us_billion'],
rich_gender_data['mat_mort_ratio'])
<matplotlib.collections.PathCollection at 0x7f66191ab890>
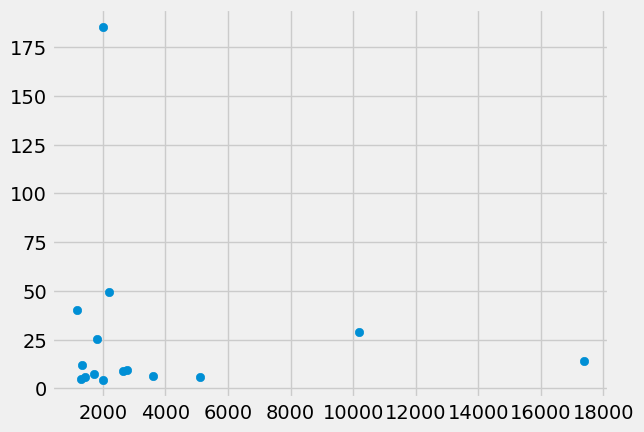