Attack or defense? An example of multiple regression#
What should you pay for, if you want to win the English Premier League?
import numpy as np
import pandas as pd
pd.set_option('mode.copy_on_write', True)
import matplotlib.pyplot as plt
# Show arrays without exponential notation, 6 digits of precision.
np.set_printoptions(suppress=True, precision=6)
<Token var=<ContextVar name='format_options' default={'edgeitems': 3, 'threshold': 1000, 'floatmode': 'maxprec', 'precision': 8, 'suppress': False, 'linewidth': 75, 'nanstr': 'nan', 'infstr': 'inf', 'sign': '-', 'formatter': None, 'legacy': 9223372036854775807, 'override_repr': None} at 0x7fa3d06db330> at 0x7fa3d6585d40>
# For minimize.
import scipy.optimize as spo
# For linregress
import scipy.stats as sps
# For running statistical models.
import statsmodels.formula.api as smf
We load the data on wage spends in the 2021-2022 English Premier League (EPL).
See the EPL dataset page for more details.
df = pd.read_csv('data/premier_league_2021.csv')
df.head()
rank | team | played | won | drawn | lost | for | against | goal_difference | points | wages_year | keeper | defense | midfield | forward | |
---|---|---|---|---|---|---|---|---|---|---|---|---|---|---|---|
0 | 1 | Manchester City | 38 | 29 | 6 | 3 | 99 | 26 | 73 | 93 | 168572 | 8892 | 60320 | 24500 | 74860 |
1 | 2 | Liverpool | 38 | 28 | 8 | 2 | 94 | 26 | 68 | 92 | 148772 | 14560 | 46540 | 47320 | 40352 |
2 | 3 | Chelsea | 38 | 21 | 11 | 6 | 76 | 33 | 43 | 74 | 187340 | 12480 | 51220 | 51100 | 72540 |
3 | 4 | Tottenham Hotspur | 38 | 22 | 5 | 11 | 69 | 40 | 29 | 71 | 110416 | 6760 | 29516 | 30680 | 43460 |
4 | 5 | Arsenal | 38 | 22 | 3 | 13 | 61 | 48 | 13 | 69 | 118074 | 8400 | 37024 | 27300 | 45350 |
Notice that we have the total yearly wage bill for each team, as well as the individual spends on the keeper, defense, midfield and forwards.
There are 20 EPL teams.
len(df)
20
The data frame describe method gives useful statistics for all the numerical columns.
df.describe()
rank | played | won | drawn | lost | for | against | goal_difference | points | wages_year | keeper | defense | midfield | forward | |
---|---|---|---|---|---|---|---|---|---|---|---|---|---|---|
count | 20.00000 | 20.0 | 20.000000 | 20.0000 | 20.000000 | 20.000000 | 20.000000 | 20.000000 | 20.00000 | 20.000000 | 20.000000 | 20.000000 | 20.000000 | 20.00000 |
mean | 10.50000 | 38.0 | 14.600000 | 8.8000 | 14.600000 | 53.550000 | 53.550000 | 0.000000 | 52.60000 | 91201.650000 | 7826.900000 | 28240.350000 | 20573.600000 | 34560.80000 |
std | 5.91608 | 0.0 | 6.762124 | 3.5333 | 6.451438 | 19.454332 | 16.223683 | 33.829293 | 19.34561 | 56966.366691 | 6188.993744 | 15652.908004 | 13186.983145 | 26175.61029 |
min | 1.00000 | 38.0 | 5.000000 | 3.0000 | 2.000000 | 23.000000 | 26.000000 | -61.000000 | 22.00000 | 28606.000000 | 2080.000000 | 8686.000000 | 3980.000000 | 6280.00000 |
25% | 5.75000 | 38.0 | 10.500000 | 6.0000 | 11.750000 | 42.000000 | 43.750000 | -20.000000 | 39.75000 | 47872.500000 | 3065.000000 | 14925.000000 | 11747.500000 | 17805.50000 |
50% | 10.50000 | 38.0 | 13.000000 | 8.0000 | 14.500000 | 49.000000 | 53.500000 | -2.000000 | 50.00000 | 75622.000000 | 7410.000000 | 26718.000000 | 16386.000000 | 26410.00000 |
75% | 15.25000 | 38.0 | 17.250000 | 11.0000 | 18.000000 | 61.250000 | 63.000000 | 10.000000 | 60.75000 | 112330.500000 | 9462.500000 | 32890.000000 | 28145.000000 | 41129.00000 |
max | 20.00000 | 38.0 | 29.000000 | 15.0000 | 27.000000 | 99.000000 | 84.000000 | 73.000000 | 93.00000 | 238780.000000 | 28600.000000 | 60480.000000 | 51100.000000 | 112780.00000 |
Notice mean (and sum) goal_difference
is 0. This must be so, because every
goal counts 1 positive for the scoring team, and 1 negative for the other team.
The shape attribute of the data frame gives the number of rows and the number of columns.
df.shape
(20, 15)
We are interested in whether the wage spend can predict the goal difference. Maybe if you pay more, you score more goals and keep more goals out.
df.plot.scatter(x='wages_year', y='goal_difference')
# We show the y-axis so we can estimate the intercept.
plt.xlim(0, 300000)
(0.0, 300000.0)
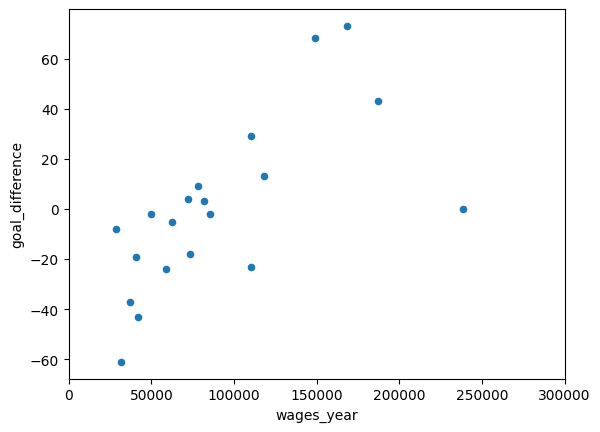
We want a best-fit line to these data.
We can use a cost-function to tell use the quality of the line. Low cost corresponds to a good line.
We use the usual sum-of-squared errors as the cost.
def sse_cost(params, x, y):
""" Sum of squared error cost function
"""
b, c = params
y_hat = b * x + c
errors = y - y_hat
return np.sum(errors ** 2)
Here are some estimates for the intercept and slope, by eye-balling the graph.
guessed_intercept = -40
# Goal difference increases by about 20 for a £50,000 increase in spend.
# Slope is rise (in y) divided by run (over x).
guessed_slope = 20 / 50000
guessed_slope
0.0004
We use minimize and the 'powell'
method to find the values for slope and intercept (params
) that give the lowest values for the cost function.
res = spo.minimize(sse_cost, [guessed_intercept, guessed_slope],
method='powell',
args=(df['wages_year'], df['goal_difference']))
res
message: Optimization terminated successfully.
success: True
status: 0
fun: 12070.170784478678
x: [ 3.728e-04 -3.360e+01]
nit: 34
direc: [[ 5.352e-07 1.264e-01]
[ 8.769e-07 8.421e-02]]
nfev: 833
These are the parameters (the slope and the intercept):
res.x
array([ 0.000373, -33.601334])
If we run linregress
, this uses the mathematics of sum-of-squares to get the
minimizing slope and intercept.
lr_res = sps.linregress(df['wages_year'], df['goal_difference'])
lr_res
LinregressResult(slope=np.float64(0.00039689691255513594), intercept=np.float64(-36.197653304934114), rvalue=np.float64(0.6683490305377195), pvalue=np.float64(0.0012767215478024995), stderr=np.float64(0.0001041171418862387), intercept_stderr=np.float64(11.11698541165042))
We suspect that minimize
was less accurate than sps.linregress
; the very
small slope may mean that minimize
gives up searching for the exact correct
slope when it has got very close.
Here’s the cost function value from the parameters from minimize
.
sse_cost(res.x, df['wages_year'], df['goal_difference'])
np.float64(12070.170784478678)
The parameters from sps.linregress
are slightly better — they give a somewhat lower value for the cost function.
sse_cost([lr_res.slope, lr_res.intercept], df['wages_year'], df['goal_difference'])
np.float64(12031.163363559293)
In data science practice, we often use these least-squares cost functions, and we can use convenient libraries for these calculations. statsmodels
is a useful library for these general models.
Using statsmodels
, we first create a model, that encodes the relationship
we’re investigating, along with the data.
sm_model = smf.ols('goal_difference ~ wages_year', data=df)
sm_model
<statsmodels.regression.linear_model.OLS at 0x7fa39854b710>
Once we have specified this model, we can use the .fit
method of the new
model to calculate the least-squares parameters.
sm_fit = sm_model.fit()
sm_fit
<statsmodels.regression.linear_model.RegressionResultsWrapper at 0x7fa37f780b90>
Finally, we can use the .summary
method of the fit result, to show a detailed
display of the parameters and other calculations from the fit.
sm_fit.summary()
Dep. Variable: | goal_difference | R-squared: | 0.447 |
---|---|---|---|
Model: | OLS | Adj. R-squared: | 0.416 |
Method: | Least Squares | F-statistic: | 14.53 |
Date: | Tue, 03 Sep 2024 | Prob (F-statistic): | 0.00128 |
Time: | 10:05:32 | Log-Likelihood: | -92.374 |
No. Observations: | 20 | AIC: | 188.7 |
Df Residuals: | 18 | BIC: | 190.7 |
Df Model: | 1 | ||
Covariance Type: | nonrobust |
coef | std err | t | P>|t| | [0.025 | 0.975] | |
---|---|---|---|---|---|---|
Intercept | -36.1977 | 11.117 | -3.256 | 0.004 | -59.554 | -12.842 |
wages_year | 0.0004 | 0.000 | 3.812 | 0.001 | 0.000 | 0.001 |
Omnibus: | 1.288 | Durbin-Watson: | 1.194 |
---|---|---|---|
Prob(Omnibus): | 0.525 | Jarque-Bera (JB): | 0.487 |
Skew: | -0.375 | Prob(JB): | 0.784 |
Kurtosis: | 3.142 | Cond. No. | 2.05e+05 |
Notes:
[1] Standard Errors assume that the covariance matrix of the errors is correctly specified.
[2] The condition number is large, 2.05e+05. This might indicate that there are
strong multicollinearity or other numerical problems.
Defense or attack?#
Here’s a reminder of the columns of data we have.
df.head()
rank | team | played | won | drawn | lost | for | against | goal_difference | points | wages_year | keeper | defense | midfield | forward | |
---|---|---|---|---|---|---|---|---|---|---|---|---|---|---|---|
0 | 1 | Manchester City | 38 | 29 | 6 | 3 | 99 | 26 | 73 | 93 | 168572 | 8892 | 60320 | 24500 | 74860 |
1 | 2 | Liverpool | 38 | 28 | 8 | 2 | 94 | 26 | 68 | 92 | 148772 | 14560 | 46540 | 47320 | 40352 |
2 | 3 | Chelsea | 38 | 21 | 11 | 6 | 76 | 33 | 43 | 74 | 187340 | 12480 | 51220 | 51100 | 72540 |
3 | 4 | Tottenham Hotspur | 38 | 22 | 5 | 11 | 69 | 40 | 29 | 71 | 110416 | 6760 | 29516 | 30680 | 43460 |
4 | 5 | Arsenal | 38 | 22 | 3 | 13 | 61 | 48 | 13 | 69 | 118074 | 8400 | 37024 | 27300 | 45350 |
Our question now is — should we spend on defense, or spend on attack (forwards)?
Here’s the plot of defense spending as a function of goal difference.
df.plot.scatter(x='defense', y='goal_difference')
<Axes: xlabel='defense', ylabel='goal_difference'>
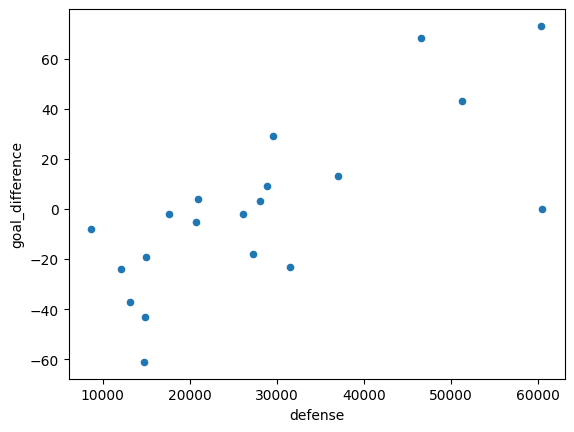
And the relationship of forward
spending and goal difference.
df.plot.scatter(x='forward', y='goal_difference')
<Axes: xlabel='forward', ylabel='goal_difference'>
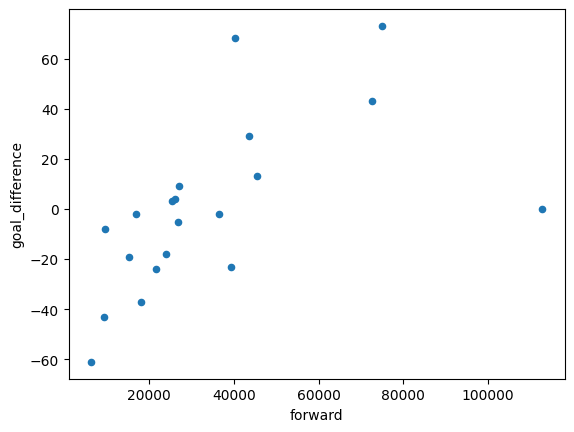
Notice that both, on their own, have strongly positive relationships.
Here we put both on the same plot.
plt.scatter(df['forward'], df['goal_difference'], label='Forward')
plt.scatter(df['defense'], df['goal_difference'], label='Defense')
plt.legend()
<matplotlib.legend.Legend at 0x7fa37d6432d0>
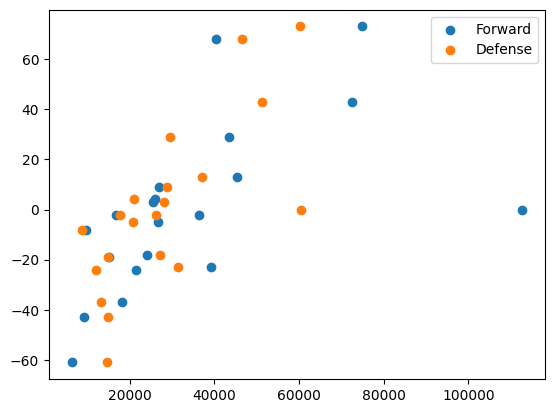
Here we calculate the simple regression models for each. Notice the chained execution for calculating the model, then the fit, and then the summary.
smf.ols('goal_difference ~ defense', data=df).fit().summary()
Dep. Variable: | goal_difference | R-squared: | 0.549 |
---|---|---|---|
Model: | OLS | Adj. R-squared: | 0.524 |
Method: | Least Squares | F-statistic: | 21.94 |
Date: | Tue, 03 Sep 2024 | Prob (F-statistic): | 0.000185 |
Time: | 10:05:32 | Log-Likelihood: | -90.322 |
No. Observations: | 20 | AIC: | 184.6 |
Df Residuals: | 18 | BIC: | 186.6 |
Df Model: | 1 | ||
Covariance Type: | nonrobust |
coef | std err | t | P>|t| | [0.025 | 0.975] | |
---|---|---|---|---|---|---|
Intercept | -45.2366 | 10.976 | -4.121 | 0.001 | -68.297 | -22.176 |
defense | 0.0016 | 0.000 | 4.684 | 0.000 | 0.001 | 0.002 |
Omnibus: | 1.906 | Durbin-Watson: | 1.356 |
---|---|---|---|
Prob(Omnibus): | 0.386 | Jarque-Bera (JB): | 1.197 |
Skew: | -0.597 | Prob(JB): | 0.550 |
Kurtosis: | 2.891 | Cond. No. | 6.75e+04 |
Notes:
[1] Standard Errors assume that the covariance matrix of the errors is correctly specified.
[2] The condition number is large, 6.75e+04. This might indicate that there are
strong multicollinearity or other numerical problems.
smf.ols('goal_difference ~ forward', data=df).fit().summary()
Dep. Variable: | goal_difference | R-squared: | 0.332 |
---|---|---|---|
Model: | OLS | Adj. R-squared: | 0.295 |
Method: | Least Squares | F-statistic: | 8.934 |
Date: | Tue, 03 Sep 2024 | Prob (F-statistic): | 0.00787 |
Time: | 10:05:32 | Log-Likelihood: | -94.262 |
No. Observations: | 20 | AIC: | 192.5 |
Df Residuals: | 18 | BIC: | 194.5 |
Df Model: | 1 | ||
Covariance Type: | nonrobust |
coef | std err | t | P>|t| | [0.025 | 0.975] | |
---|---|---|---|---|---|---|
Intercept | -25.7248 | 10.698 | -2.405 | 0.027 | -48.200 | -3.250 |
forward | 0.0007 | 0.000 | 2.989 | 0.008 | 0.000 | 0.001 |
Omnibus: | 1.089 | Durbin-Watson: | 1.010 |
---|---|---|---|
Prob(Omnibus): | 0.580 | Jarque-Bera (JB): | 0.161 |
Skew: | 0.120 | Prob(JB): | 0.923 |
Kurtosis: | 3.369 | Cond. No. | 7.23e+04 |
Notes:
[1] Standard Errors assume that the covariance matrix of the errors is correctly specified.
[2] The condition number is large, 7.23e+04. This might indicate that there are
strong multicollinearity or other numerical problems.
Now we are interesting in taking both defense and forward spending into account at the same time.
This is multiple regression.
Notice that in multiple regression, we calculate the fitted \(\hat{\vec{y}}\) values by adding to together the components due to the first predictor, and that due to the second predictor, and the intercept.
def sse_multi(params, X, y):
""" Multiple regression sum of squared error
Parameters
----------
params : slopes and intercept
X : array
2D array of predictor columns.
y : array
1D array of outcome vector.
Returns
-------
cfv : float
Cost function value
"""
n, p = X.shape
y_hat = np.zeros(n)
for col_no in range(p): # Go through each column.
col = X[:, col_no] # Get the relevant column from X
fit_for_this_col = col * params[col_no] # Multiply by corresponding parameter
y_hat = y_hat + fit_for_this_col
y_hat = y_hat + params[-1] # Add the intercept
errors = y - y_hat
return np.sum(errors ** 2)
We compile the 2D array containing the two predictor columns, by taking two columns out of the data frame, and converting to an array.
X = np.array(df[['defense', 'forward']])
X
array([[ 60320, 74860],
[ 46540, 40352],
[ 51220, 72540],
[ 29516, 43460],
[ 37024, 45350],
[ 60480, 112780],
[ 28860, 27040],
[ 28040, 25440],
[ 17640, 16790],
[ 20736, 26820],
[ 27276, 24040],
[ 20930, 26000],
[ 8686, 9620],
[ 26160, 36470],
[ 12137, 21530],
[ 31512, 39310],
[ 13150, 18144],
[ 14940, 15110],
[ 14880, 9280],
[ 14760, 6280]])
Next we use minimize
to find the three parameters that minimize the cost
function. We found that both powell
and the default methods gave warnings about failing to reach good results, so we tried the nelder-mead
method.
min_res_2 = spo.minimize(sse_multi, [0.001, 0.001, -36],
method='nelder-mead',
args=(X, df['goal_difference']))
min_res_2
message: Optimization terminated successfully.
success: True
status: 0
fun: 8270.703854709001
x: [ 2.933e-03 -8.665e-04 -5.288e+01]
nit: 107
nfev: 192
final_simplex: (array([[ 2.933e-03, -8.665e-04, -5.288e+01],
[ 2.933e-03, -8.665e-04, -5.288e+01],
[ 2.933e-03, -8.665e-04, -5.288e+01],
[ 2.933e-03, -8.665e-04, -5.288e+01]]), array([ 8.271e+03, 8.271e+03, 8.271e+03, 8.271e+03]))
Here are the parameters:
slope for
defense
slope for
attack
intercept.
min_res_2.x
array([ 0.002933, -0.000867, -52.875186])
We can do the same parameter estimating using statsmodels
in our case, because we are using the standard least-squares cost function.
fitted = smf.ols('goal_difference ~ defense + forward', data=df).fit()
fitted.summary()
Dep. Variable: | goal_difference | R-squared: | 0.620 |
---|---|---|---|
Model: | OLS | Adj. R-squared: | 0.575 |
Method: | Least Squares | F-statistic: | 13.85 |
Date: | Tue, 03 Sep 2024 | Prob (F-statistic): | 0.000270 |
Time: | 10:05:32 | Log-Likelihood: | -88.626 |
No. Observations: | 20 | AIC: | 183.3 |
Df Residuals: | 17 | BIC: | 186.2 |
Df Model: | 2 | ||
Covariance Type: | nonrobust |
coef | std err | t | P>|t| | [0.025 | 0.975] | |
---|---|---|---|---|---|---|
Intercept | -52.8752 | 11.236 | -4.706 | 0.000 | -76.581 | -29.169 |
defense | 0.0029 | 0.001 | 3.587 | 0.002 | 0.001 | 0.005 |
forward | -0.0009 | 0.000 | -1.772 | 0.094 | -0.002 | 0.000 |
Omnibus: | 1.441 | Durbin-Watson: | 1.303 |
---|---|---|---|
Prob(Omnibus): | 0.486 | Jarque-Bera (JB): | 1.207 |
Skew: | -0.548 | Prob(JB): | 0.547 |
Kurtosis: | 2.501 | Cond. No. | 1.21e+05 |
Notes:
[1] Standard Errors assume that the covariance matrix of the errors is correctly specified.
[2] The condition number is large, 1.21e+05. This might indicate that there are
strong multicollinearity or other numerical problems.
The found parameters are almost exactly what we found with minimize
.
fitted.params
Intercept -52.875175
defense 0.002933
forward -0.000867
dtype: float64
The parameters make us think that we gain very little — or even lose — by extra spending on forwards, given a particular level of spending on defense.
Another way of looking at this is to first remove (“regress out”) the effect of spending on defense, leaving the errors (AKA residuals). The residuals are what remains of goal difference after we have done the best job we can (in the least-squares sense) of removing the effect of spending on defense.
defense_only_fit = smf.ols('goal_difference ~ defense', data=df).fit()
defense_only_fit.params
Intercept -45.236577
defense 0.001602
dtype: float64
Here we calculate residuals (errors) left over after using the fit from the defense-only model.
y_hat = defense_only_fit.params['defense'] * df['defense'] + defense_only_fit.params['Intercept']
errors = df['goal_difference'] - y_hat
errors
0 21.613471
1 38.686853
2 6.190233
3 26.956610
4 -1.070019
5 -51.642824
6 8.007419
7 3.320929
8 14.980085
9 7.020783
10 -16.455264
11 15.710025
12 23.322978
13 1.332392
14 1.795021
15 -28.240666
16 -12.827644
17 2.305058
18 -21.598831
19 -39.406610
dtype: float64
In fact Statsmodels is kind enough to calculate these for us, by default, in the resid
attribute of the fit.
defense_only_fit.resid
0 21.613471
1 38.686853
2 6.190233
3 26.956610
4 -1.070019
5 -51.642824
6 8.007419
7 3.320929
8 14.980085
9 7.020783
10 -16.455264
11 15.710025
12 23.322978
13 1.332392
14 1.795021
15 -28.240666
16 -12.827644
17 2.305058
18 -21.598831
19 -39.406610
dtype: float64
We can take the residual — left over — values, and put them back into the data frame, so Statmodels can do another fit.
df['without_defense'] = fitted.resid
df
rank | team | played | won | drawn | lost | for | against | goal_difference | points | wages_year | keeper | defense | midfield | forward | without_defense | |
---|---|---|---|---|---|---|---|---|---|---|---|---|---|---|---|---|
0 | 1 | Manchester City | 38 | 29 | 6 | 3 | 99 | 26 | 73 | 93 | 168572 | 8892 | 60320 | 24500 | 74860 | 13.837431 |
1 | 2 | Liverpool | 38 | 28 | 8 | 2 | 94 | 26 | 68 | 92 | 148772 | 14560 | 46540 | 47320 | 40352 | 19.349313 |
2 | 3 | Chelsea | 38 | 21 | 11 | 6 | 76 | 33 | 43 | 74 | 187340 | 12480 | 51220 | 51100 | 72540 | 8.515417 |
3 | 4 | Tottenham Hotspur | 38 | 22 | 5 | 11 | 69 | 40 | 29 | 71 | 110416 | 6760 | 29516 | 30680 | 43460 | 32.970125 |
4 | 5 | Arsenal | 38 | 22 | 3 | 13 | 61 | 48 | 13 | 69 | 118074 | 8400 | 37024 | 27300 | 45350 | -3.411476 |
5 | 6 | Manchester United | 38 | 16 | 10 | 12 | 57 | 57 | 0 | 58 | 238780 | 28600 | 60480 | 36920 | 112780 | -26.773404 |
6 | 7 | West Ham United | 38 | 16 | 8 | 14 | 60 | 51 | 9 | 56 | 77936 | 11856 | 28860 | 10180 | 27040 | 0.665784 |
7 | 8 | Leicester City | 38 | 14 | 10 | 14 | 62 | 59 | 3 | 52 | 81590 | 10040 | 28040 | 18070 | 25440 | -4.315766 |
8 | 9 | Brighton and Hove Albion | 38 | 12 | 15 | 11 | 42 | 44 | -2 | 51 | 49820 | 3120 | 17640 | 12270 | 16790 | 13.689769 |
9 | 10 | Wolverhampton Wanderers | 38 | 15 | 6 | 17 | 38 | 43 | -5 | 51 | 62756 | 2120 | 20736 | 13080 | 26820 | 10.301066 |
10 | 11 | Newcastle United | 38 | 13 | 10 | 15 | 44 | 62 | -18 | 49 | 73308 | 4940 | 27276 | 17052 | 24040 | -24.288248 |
11 | 12 | Crystal Palace | 38 | 11 | 15 | 12 | 50 | 46 | 4 | 48 | 71910 | 6000 | 20930 | 18980 | 26000 | 18.021561 |
12 | 13 | Brentford | 38 | 13 | 7 | 18 | 48 | 56 | -8 | 46 | 28606 | 2080 | 8686 | 8220 | 9620 | 27.736951 |
13 | 14 | Aston Villa | 38 | 13 | 6 | 19 | 52 | 54 | -2 | 45 | 85330 | 8320 | 26160 | 14380 | 36470 | 5.755570 |
14 | 15 | Southampton | 38 | 9 | 13 | 16 | 43 | 67 | -24 | 40 | 58657 | 9270 | 12137 | 15720 | 21530 | 11.936166 |
15 | 16 | Everton | 38 | 11 | 6 | 21 | 43 | 66 | -23 | 39 | 110202 | 8060 | 31512 | 31320 | 39310 | -28.479761 |
16 | 17 | Leeds United | 38 | 9 | 11 | 18 | 42 | 79 | -37 | 38 | 37354 | 2080 | 13150 | 3980 | 18144 | -6.968775 |
17 | 18 | Burnley | 38 | 7 | 14 | 17 | 34 | 53 | -19 | 35 | 40830 | 2900 | 14940 | 7880 | 15110 | 3.152527 |
18 | 19 | Watford | 38 | 6 | 5 | 27 | 34 | 77 | -43 | 23 | 42030 | 2580 | 14880 | 15290 | 9280 | -25.723314 |
19 | 20 | Norwich City | 38 | 5 | 7 | 26 | 23 | 84 | -61 | 22 | 31750 | 3480 | 14760 | 7230 | 6280 | -45.970937 |
Now we look to see if the forward
spending can predict what is left over, after taking into account the effect of defense spending on goal difference:
smf.ols('without_defense ~ forward', data=df).fit().summary()
Dep. Variable: | without_defense | R-squared: | 0.000 |
---|---|---|---|
Model: | OLS | Adj. R-squared: | -0.056 |
Method: | Least Squares | F-statistic: | 0.000 |
Date: | Tue, 03 Sep 2024 | Prob (F-statistic): | 1.00 |
Time: | 10:05:32 | Log-Likelihood: | -88.626 |
No. Observations: | 20 | AIC: | 181.3 |
Df Residuals: | 18 | BIC: | 183.2 |
Df Model: | 1 | ||
Covariance Type: | nonrobust |
coef | std err | t | P>|t| | [0.025 | 0.975] | |
---|---|---|---|---|---|---|
Intercept | -1.288e-14 | 8.071 | -1.6e-15 | 1.000 | -16.956 | 16.956 |
forward | -1.335e-18 | 0.000 | -7.11e-15 | 1.000 | -0.000 | 0.000 |
Omnibus: | 1.441 | Durbin-Watson: | 1.303 |
---|---|---|---|
Prob(Omnibus): | 0.486 | Jarque-Bera (JB): | 1.207 |
Skew: | -0.548 | Prob(JB): | 0.547 |
Kurtosis: | 2.501 | Cond. No. | 7.23e+04 |
Notes:
[1] Standard Errors assume that the covariance matrix of the errors is correctly specified.
[2] The condition number is large, 7.23e+04. This might indicate that there are
strong multicollinearity or other numerical problems.
It appears that this two-stage procedure gives a similar result to the one we saw before — once we have accounted for spending on defense, spending on forwards is no longer important, in these models.